Numerical estimation of parameters in mathematical models for pest control
DOI:
https://doi.org/10.19136/jobs.a10n29.6375Palabras clave:
Equilibrium points, Multi-stept method, Parameter estimation, Spux, CommnesalistResumen
In this work we focused on the estimation of the parameters belonging to a mathematical model for the interaction between three species (plant, plague and bio control agent), with the goal to guaranty the survival of all them. A qualitative analysis of the model is carried out to show the dynamic of it. As well, the model is solved by using a numerical scheme based on multi step method. The obtained code is coupled with the Spux framework to estimate, by stochastic simulations, the unknown parameters of the model. Numerical results show that Spux gives a correct approximation of the parameters because the model simulations with the approximated parameters are in good agreement with the data.
Referencias
P. A. Abrams and L.R. Ginzburg. “The nature of predation: prey dependent, ratio dependent or neither?” Trends in Ecology & Evolution, 15(8):337–341, 2000. https://doi.org/10.1016/S0169-5347(00)01908-X
C. Albert, H. R. K´’unsch, and A. Scheidegger. “A simulated annealing approach to approximate bayes computations”. Statistics and Computing, 25:1217–1232, 2012. https://api.semanticscholar.org/CorpusID:8239929
Roger Arditi and Lev R. Ginzburg. “Coupling in predator-prey dynamics: Ratio-dependence”. Journal of Theoretical Biology, 139(3):311–326, 1989. https://doi.org/10.1016/S0022-5193(89)80211-5
J. C. Butcher. Numerical Differential Equation Methods, chapter 2, pages 55–142. John Wiley & Sons, Ltd, 2016. https://doi.org/10.1002/9781119121534.ch2
J. C. Butcher. Runge - Kutta Methods, chapter 3, pages 143–331. John Wiley & Sons, Ltd, 2016. https://doi.org/10.1002/9781119121534.ch3
A.M. Ellison. “Bayesian inference in ecology”. Ecology Letters, 7(6):509–520, 2004. https://doi.org/10.1111/j.1461-0248.2004.00603.x
D. Gamerman and H. F. Lopes. Markov Chain Monte Carlo Stochastic Simulation for Bayesian Inference, Second Edition. Chapman & Hall/CRC, 2006.
D. Gamerman and H.F. Lopes. Markov Chain Monte Carlo: Stochastic Simulation for Bayesian Inference, Second Edition. Chapman & Hall/CRC Texts in Statistical Science. Taylor & Francis, 2006. https://books.google.com.mx/books?id=yPvECi L3bwC
E. Hairer, S.P. Nørsett, and G. Wanner. “Solving Ordinary Differential Equations I: Nonstiff Problems”. Springer Series in Computational Mathematics. Springer Berlin Heidelberg, 2008. https://books.google.com.mx/books?id=F93u7VcSRyYC
W. K. Hastings. “Monte carlo sampling methods using markov chains and their applications”. Biometrika, 57(1):97–109, 1970. http://www.jstor.org/stable/2334940
M. F. Jim´enez, G. Ble, and M. Falconi. “A biocontrol agent as a commensal in a plant-pest interaction & nbsp”. ECOLOGICAL MODELLING, 468, 2022. https://ideas.repec.org/a/eee/ecomod/v468y2022ics0304380022000758.html
J. Peinado, J. Ib´an˜ez, E. Arias, and V. Hernández. ‘Adams bashforth and adams moulton methods for solving differential riccati equations”. Computers & Mathematics with Applications, 60(11):3032–3045, 2010. https://doi.org/10.1016/j.camwa.2010.10.002
E. Rukmangadachari. Mathematical Methods. Dorling Kindersley, 2009. https://books.google.com.mx/books?id=Vju6XxmlGcUC
A. Safin, D. Bouffard, F. Ozdemir, C. L. Ram´on, J. Runnalls, F. Georgatos, C. Minaudo, and J. Sukys. “A bayesian data assimilation framework for lake 3d hydrodynamic models with a physics-preserving particle filtering method using spux-mitgcm v1”. Geoscientific Model Development, 15(20):7715–7730, 2022.
Scientific Computing Group at Eawag and SIS ID at ETH Zurich. spux Documentation, Release 0.4.0, November 2019.
J. Sukys and B Bacci. “Spux framework: a scalable package for bayesian uncertainty quantification and propagation”, 2021.
J. Sukys and M. Kattwinkel. “Spux: Scalable particle markov chain monte carlo for uncertainty quantification in stochastic ecological models”, 2017. https://doi.org/10.48550/arXiv.2105.05969
A. Tutueva, T. Karimov, and D. Butusov. “Semi-implicit and semi-explicit adams-bashforth-moulton methods”. Mathematics, 8(5), 2020. https://www.mdpi.com/2227-7390/8/5/780
J.M. Zelle. Python Programming: An Introduction to Computer Science. Franklin Beedle Series. Franklin, Beedle, 2004. https://books.google.com.mx/books?id=aJQILlLxRmAC
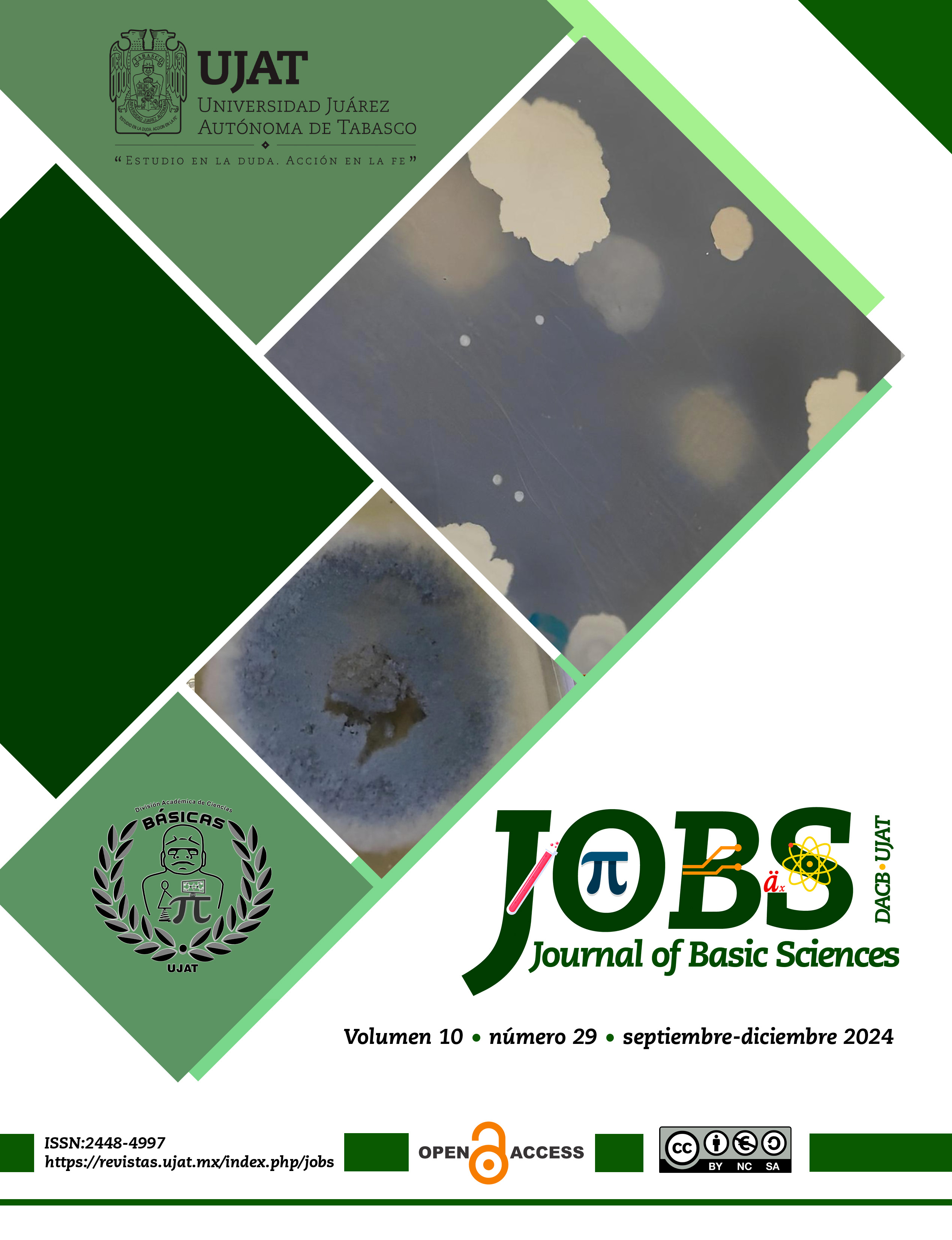
Descargas
Publicado
Número
Sección
Licencia
Derechos de autor 2024 Journal of Basic Sciences

Esta obra está bajo una licencia internacional Creative Commons Atribución-NoComercial-CompartirIgual 4.0.
Usted es libre de:
- Compartir — copiar y redistribuir el material en cualquier medio o formato
- La licenciante no puede revocar estas libertades en tanto usted siga los términos de la licencia
Bajo los siguientes términos:
- Atribución — Usted debe dar crédito de manera adecuada , brindar un enlace a la licencia, e indicar si se han realizado cambios . Puede hacerlo en cualquier forma razonable, pero no de forma tal que sugiera que usted o su uso tienen el apoyo de la licenciante.
- NoComercial — Usted no puede hacer uso del material con propósitos comerciales .
- SinDerivadas — Si remezcla, transforma o crea a partir del material, no podrá distribuir el material modificado.
- No hay restricciones adicionales — No puede aplicar términos legales ni medidas tecnológicas que restrinjan legalmente a otras a hacer cualquier uso permitido por la licencia.
Avisos:
No tiene que cumplir con la licencia para elementos del materiale en el dominio público o cuando su uso esté permitido por una excepción o limitación aplicable.
No se dan garantías. La licencia podría no darle todos los permisos que necesita para el uso que tenga previsto. Por ejemplo, otros derechos como publicidad, privacidad, o derechos morales pueden limitar la forma en que utilice el material.